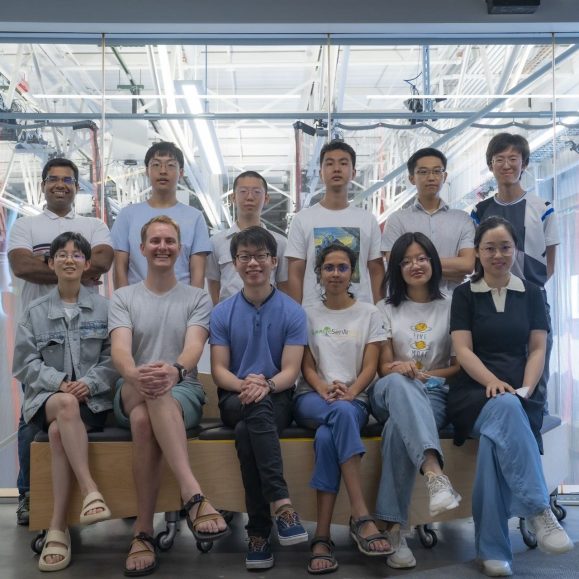
REALM
Welcome to REALM!
Welcome to the Reliable Autonomous Systems Lab at MIT (REALM)! We design, analyze and verify safe control systems. Our work lies at the intersection of control theory, machine learning, and formal methods, with a focus on safety in systems with nonlinear, high-dimensional and difficult-to-model dynamics.
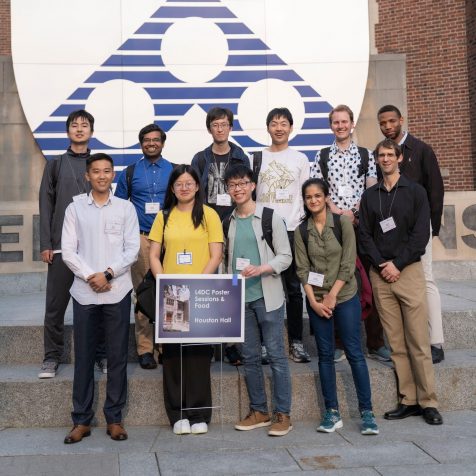
Recent News
- [Jan'24]: Charles got the best presenter award for the 29th Annual LIDS Student Conference (Autonomy, Control, & Applications session)
- [Nov' 23]: Charles presented at the MIT Energy & Climate Club Seminar
- [Oct' 23]: One paper accepted at EMNLP
- [Oct' 23]: Charles presented at NAPS 2023
- [Aug' 23]: Yue got Amazon Robotics Fellowship
- [Jun' 23]: Mingxin got MathWorks Fellowship
- [Mar' 23]: One paper and workshop accepted at RSS 2023
- [Mar' 23]: Two papers (one oral) accepted at L4DC 2023
- [Jan' 23]: Chuchu got AFOSR YIP Award and NSF CAREER Award
- [Jan' 23]: Two papers accepted at ICRA
- [Oct' 22]: Chuchu invited present at NCCR Symposium in Switzerland as part of IEEE CSS Day
- [Sept' 22]: Kunal Garg, Oswin So, Yongchao Chen, Mingxin Yu, Ruixiao Yang and Rujul Gandhi join REALM
- [Jun'22]: Chuchu gives a Keynote seminar at L4DC conference at Stanford University
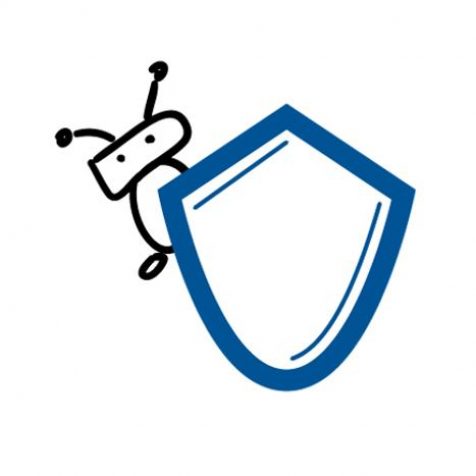
Our Missions
We look at autonomy through two complementary points of view:
- Given an autonomous system, how do we know whether it will accomplish its mission safely?
- How can we use safety considerations to design better autonomous systems?
Currently, we are thinking a lot about how these questions can apply to learning-enabled autonomy but we are also interested in exploring these questions more broadly.